What’s a Unique Challenge You’ve Faced Working With Machine Learning Models for Computational Linguistics?
Navigating the intricate web of machine learning in the realm of computational linguistics is akin to decoding an ancient manuscript without a reference guide. It requires a blend of precision and intuition, a journey best undertaken by experts in the field. Amongst these trailblazers stands a CEO, unraveling the nuances of language, and a data scientist, adeptly managing and understanding linguistic disagreements. In this article, they share insights that travel from addressing language nuances right through to managing training data biases, encapsulating four unique challenges faced and conquered in this cutting-edge domain.
- Address Language Nuances
- Manage Training Data Biases
- Speak The Language Of Freight
- Navigate Linguistic Disagreements
Address Language Nuances
A unique challenge often encountered in computational linguistics when working with machine-learning models is dealing with the nuances and variability of natural language. Language is inherently complex and context-dependent, which makes it difficult for models to accurately interpret and generate text that aligns with human expectations.
For instance, machine-learning models can struggle with polysemy, where a single word has multiple meanings depending on the context. Additionally, capturing subtle nuances like sarcasm or regional dialects can be particularly challenging. To address these issues, it's crucial to use diverse and extensive datasets and employ advanced techniques such as contextual embeddings (e.g., BERT, GPT) to better understand and generate human-like text.
Manage Training Data Biases
A unique challenge I've faced with machine-learning models in computational linguistics is managing biases in training data. I recommend that business leaders actively audit their datasets for diversity and representation, as this not only enhances model performance but also builds user trust.
In developing the Christian Companion App, we encountered issues where our model struggled with nuanced biblical language due to a dataset that favored certain translations. This prompted us to seek out a broader range of biblical texts, ensuring various interpretations were included, which significantly improved our model's accuracy.
To address this challenge, I advise conducting a thorough analysis of your data sources to identify biases and actively incorporating underrepresented voices. Engaging with linguistics experts and community feedback can also validate and refine your model's outputs.
This strategy proved effective for us; after refining our model, we saw a noticeable increase in user engagement and satisfaction. Addressing bias isn't just ethical—it's essential for creating relevant, high-quality AI solutions that resonate with users.
Speak The Language Of Freight
We're pioneering a monumental goal within our business, Stargo, and it has come with very unique challenges. Our GenAI suite of software is built on an LLM that speaks "Freight." It's been decades of working with our proprietary LLM to teach it the language of shipping, across unstructured data formats and types, and contextual understanding within stakeholder exchanges of that data—down to the very inbox, the emails between shipper and forwarder. The logistics industry has so much unstructured data and in so many languages that building our LLM has been a unique challenge that very few tech businesses have taken on. We're proud of the fact that we are leading in this field despite the many technical and first-to-experience challenges we've seen.
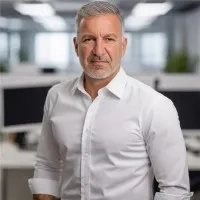
Navigate Linguistic Disagreements
A key challenge in building machine-learning models for language is getting linguists to agree, as linguistic interpretation can vary widely. Language evolves over time, meaning that what is accurate or relevant at one point might shift as new words and phrases emerge. Even at the same time, different linguists can have their own ideas about how language should be used. This makes it hard to create one "perfect" model or evaluation. It’s important to balance keeping the models adaptable while making sure they still perform well as language evolves.
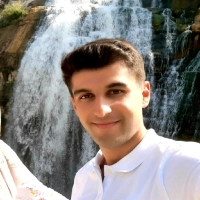