3 Overlooked Ethical Issues in Speech Technology and How to Address Them
Navigating the complex maze of ethical concerns in speech technology demands expert guidance. This article distills the wisdom of industry leaders to tackle the most pressing, yet often overlooked, challenges. It serves as a beacon for those aiming to enhance inclusivity and address accent biases by training AI on diverse datasets.
- Address Accent Bias with Diverse Data
- Train Speech AI on Diverse Datasets
- Improve Inclusivity in Speech Technology
Address Accent Bias with Diverse Data
Good day!
One ethical consideration that is often overlooked when developing or deploying speech technology is the potential for accent bias. Many speech recognition systems are primarily trained on data featuring standard accents or dominant language dialects, which can lead to inaccurate or subpar performance for users with regional accents or non-native speech patterns. This not only reduces the accessibility of such technology but also risks perpetuating systemic inequities by marginalizing those whose speech does not align with the dominant dataset. Addressing this requires diverse and representative training data to ensure fair and inclusive experiences for all users.
Developers must prioritize collecting diverse and representative datasets that capture a wide range of accents, dialects, and speech patterns to address accent bias in speech technology. This can entail sourcing data from speakers from various regions, socio-economic strata, and linguistic groups. Organizations must also ensure that their data is inclusive by working alongside linguists and community representatives. In addition, synthetic data augmentation and novel machine learning methods will improve system generalizations on weakly represented accents. It is also important to continually audit and test the systems for fairness and performance across different groups of users to identify and address biases. If successful, these practices among more stakeholders would promote equitable speech technology that is available for all to use while contributing to further study of voice AI to mitigate risk for consumers.
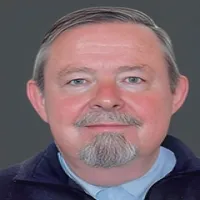
Train Speech AI on Diverse Datasets
One often overlooked ethical concern in speech technology is accent and dialect bias—where AI models struggle to accurately recognize and respond to diverse speech patterns. Many speech recognition systems are trained on limited datasets, often favoring dominant accents, which can lead to misinterpretation, exclusion, or even frustration for users with regional or non-native accents.
To address this, speech AI must be trained on diverse, representative datasets that include varied linguistic styles, tones, and speech patterns. Additionally, real-time model adaptation and user feedback loops should be incorporated to refine accuracy continuously. Transparency in AI decision-making is also crucial—users should know how speech inputs are processed and be given control over adjustments.
Speech AI should empower all users, not just a select few. Ensuring inclusivity in voice technology isn't just a technical challenge—it's a fundamental step toward equitable AI adoption in global communities.
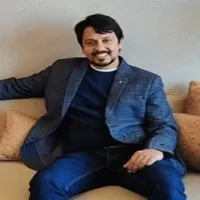
Improve Inclusivity in Speech Technology
Good day,
The accent and dialect bias is an often overlooked ethical consideration in the development and deployment of speech technology. This suggests that most speech recognition systems cannot accurately interpret speakers with diverse accents, dialects, or speech patterns, potentially creating exclusion, frustration, and even discrimination especially in critical service areas such as healthcare, customer support, and hiring processes.
Developers need to train AI models on diverse linguistic datasets and not just include white men from northern England. They should do regular bias audits to check for inequities in speech recognition performance and make fixes. They should also proactively collaborate with linguists, ethicists, and a wider range of user groups while developing technology to make it more inclusive and fair.
By prioritizing fairness and transparency and focusing our efforts on making speech technology inclusive, the potential that bias could reinforce social inequality can be avoided, and we can develop systems that truly work for all users.